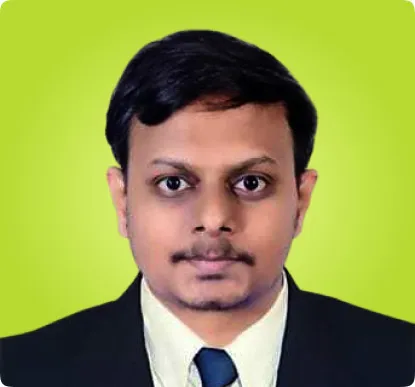
Akash
Engineering Lead
The world of artificial intelligence has been evolving rapidly since the introduction of Generative Pre-trained Transformers (GPT) by OpenAI. The emergence of Generative AI has paved the way for numerous breakthroughs, and Falcon AI, particularly Falcon LLM, has become a prominent player in this field. Developed by the UAE's Technology Innovation Institute (TII), Falcon LLM is a large language model (LLM) that is making waves with its capabilities and unique characteristics. In this step-by-step tutorial, we'll explore what sets Falcon LLM apart and how you can harness its power for various applications.
Falcon LLM: From Trillions of Tokens to Billions of Parameters
Falcon AI offers a suite of LLM models, including Falcon 180B, 40B, 7.5B, and 1.3B, each tailored to different use cases and requirements. The "B" in these model names indicates the number of parameters, which is a crucial factor in determining the model's capabilities. Falcon LLM's largest variant, the Falcon 180B, boasts a staggering 180 billion parameters and has been trained on an extensive dataset of 3.5 trillion tokens.
Key Features of Falcon LLM
- Transparent and Open Source: One of the standout features of Falcon LLM is its transparency and open-source nature. Unlike some closed-source models, Falcon LLM allows researchers and developers to access its inner workings, making it an excellent choice for those who want to understand and fine-tune the model for specific tasks.
- Rich Training Data: Falcon LLM's exceptional performance can be attributed in part to its high-quality training data. The model was trained on a diverse dataset comprising nearly five trillion tokens gathered from various sources, including public web crawls (approximately 80%), research papers, legal texts, news articles, literature, and social media conversations. This diverse data ensures that Falcon LLM possesses a wide-ranging knowledge base.
Falcon LLM Models
Now, let's delve into how Falcon LLM models are making an impact in the world of AI.
- Falcon 180B: This colossal model, with its 180 billion parameters, is currently at the top of the Hugging Face Leaderboard for pre-trained Open Large Language Models. It has showcased remarkable performance in various tasks such as reasoning, coding, proficiency assessments, and knowledge tests. Impressively, it has even outperformed competitors like Meta's LLaMA 2. While it falls just behind OpenAI's GPT-4 among closed-source models, Falcon 180B holds its ground and performs on par with Google's PaLM 2 Large, despite being half the size. 🤯
- Falcon 40B: Falcon 40B was a game-changer when it was launched, ranking as the world's top-ranked open-source AI model. With 40 billion parameters and training on a vast dataset of one trillion tokens, Falcon 40B demonstrated the power of open-source AI. For two months following its launch, it held the #1 spot on Hugging Face's leaderboard for open-source large language models. 🤯
Using Falcon LLM: A Step-by-Step Guide
- Step 1: Install the required packages
pip install --quiet torch torchvision torchaudio --index-url
https://download.pytorch.org/whl/cu117 --upgrade
pip install --quiet langchain einops accelerate transformers
bitsandbytes
from langchain import HuggingFacePipeline
from langchain import PromptTemplate, LLMChain
from transformers import AutoTokenizer, AutoModelForCausalLM
import transformers
import os
import torch
# Define Model ID
model_id = "tiiuae/falcon-7b-instruct"
# Load Tokenizer
tokenizer = AutoTokenizer.from_pretrained(model_id)
# Load Model
model = AutoModelForCausalLM.from_pretrained(model_id,
cache_dir='/opt/workspace/',
torch_dtype=torch.bfloat16, trust_remote_code=True,
device_map="auto", offload_folder="offload")
# Set PT model to inference mode
model.eval()
# Build HF Transformers pipeline
pipeline = transformers.pipeline(
"text-generation",
model=model,
tokenizer=tokenizer,
device_map="auto",
max_length=400,
do_sample=True,
top_k=10,
num_return_sequences=1,
eos_token_id=tokenizer.eos_token_id
)
question = """Translate the below text from English to French
Generative AI models are enabling us to create innovative pathways to an
exciting future of possibilities - where the only limits are of the
imagination.
Our team of AI researchers, scientists, and engineers are collaborating
to achieve innovative outcomes - from AI theory to AI technologies,
and are sharing their efforts with the wider AI community.
"""
# Test out the pipeline
pipeline(question)
[{'generated_text': "Translate the below text from English to French\n\nGenerative AI models are enabling us to create innovative pathways to an exciting future of possibilities - where the only limits are of the imagination.\n\nOur team of AI researchers, scientists, and engineers are collaborating to achieve innovative outcomes - from AI theory to AI technologies, \nand are sharing their efforts with the wider AI community.\n\n<p>Translation: \nLes modèles de l'IA génériques permettent à nous de créer des voies innovantes pour un avenir excitant d'opportunités - où les seuls limites sont de l'imagination. \nNotre équipe de chercheurs en recherche, chercheurs et ingénieurs travaillent ensemble pour atteindre des objectifs innovants - de l'IA théorique à des technologies AI - et échangent leurs connaissances avec la communauté AI."}]
sequences = pipeline(
"Create a list of 3 important things to reduce global warming"
)
for seq in sequences:
print(f"Result: {seq['generated_text']}")
Result: Create a list of 3 important things to reduce global warming
1. Conserve energy: Reduce the amount of electricity used and waste heat.
2. Drive electric or hybrid cars.
3. Plant trees and preserve forests to absorb carbon dioxide.
Alternatively we can use Langchain's PromptTemplate and LLM chain to run a query
# Setup prompt template
template = PromptTemplate(input_variables=['input'], template='{input}')
# Pass hugging face pipeline to langchain class
llm = HuggingFacePipeline(pipeline=pipeline)
# Build stacked LLM chain i.e. prompt-formatting + LLM
chain = LLMChain(llm=llm, prompt=template)
question = """Translate the below text from English to French
Generative AI models are enabling us to create innovative pathways
to an exciting future of possibilities - where the only limits are of the
imagination.
Our team of AI researchers, scientists, and engineers are collaborating
to achieve innovative outcomes - from AI theory to AI technologies,
and are sharing their efforts with the wider AI community.
"""
# Test LLMChain
response = chain.run(question)
L'IA génératrice nous permet de mettre au point de nouvelles voies à de formidables possibilités d'avenir - où seules nos limites de l'imagination s'imposent.</p> <p>Notre équipe de chercheurs en IA, scientifiques et ingénieurs travaillent ensemble pour atteindre des objectifs innovants et échanger leurs connaissances avec la communauté de l'IA.
query = "How to reach the moon?"
print(chain.run(query))
Unfortunately, currently the only way to reach the moon is through space programs run by governments or private organizations. NASA, for example, has plans to return humans to land on the Moon by 2024. However, private companies such as SpaceX and Blue Origin have also been working to develop reusable spacecraft for manned missions to the Moon.
Conclusion
Falcon LLM represents a significant advancement in the field of Generative AI. With its transparency, open-source nature, and exceptional performance, it offers a valuable tool for researchers and developers across various domains. Whether you're tackling complex reasoning tasks or building AI-powered applications, Falcon LLM's suite of models can help you unlock the potential of generative language models. As we continue to witness the evolution of AI, Falcon LLM stands as a testament to the strides being made towards achieving human-like intelligence in machines.
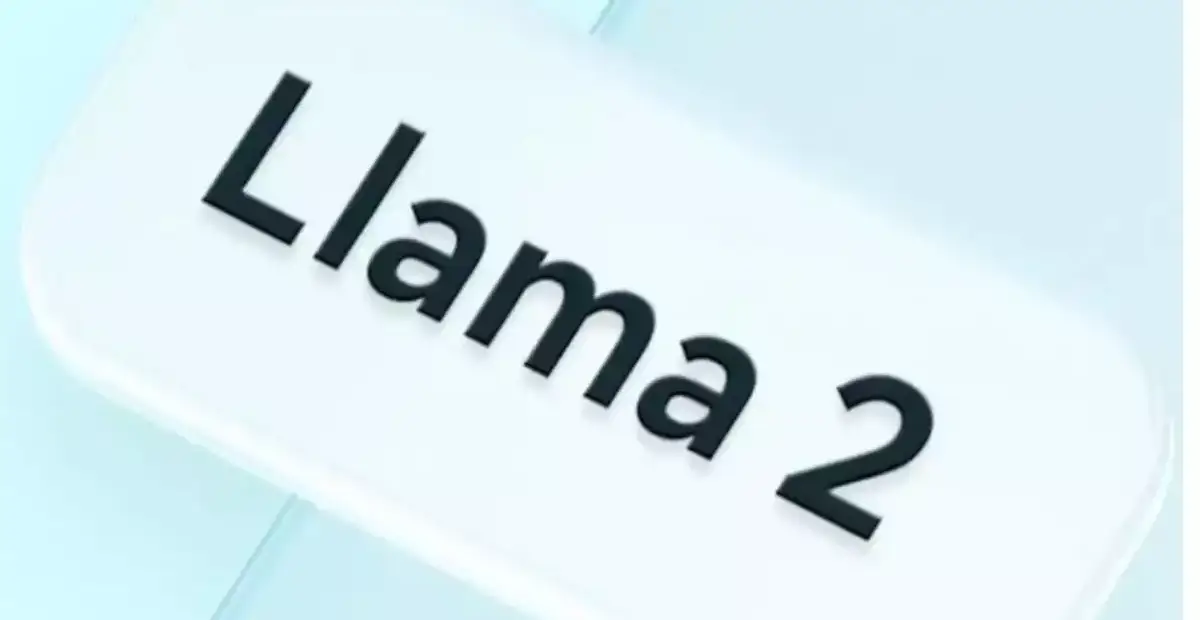
Exploring Llama 2: From Installation to Interaction
The race to create robust Generative Large Language Models (LLMs) has been heating up with the release of GPT from OpenAI. Companies are now competing to develop their own LLMs, which can be a cumbersome process involving thorough...